Analisis dan Implementasi Diagnosis Penyakit Sawit dengan Metode Convolutional Neural Network (CNN)
Abstract
Keywords
Full Text:
PDFReferences
Fajri, R. I. (2014). Identifikasi Penyakit Daun Tanaman Kelapa Sawit Menggunakan Support Vector Machine. Jurnal Teknologi Perkebunan. Retrieved from http://repository.usu.ac.id/handle/123456789/42256
Huang, B., Ou, Y., & Carley, K. M. (2018). Aspect level sentiment classification with attention-over-attention neural networks. Lecture Notes in Computer Science (Including Subseries Lecture Notes in Artificial Intelligence and Lecture Notes in Bioinformatics), 10899 LNCS, 197–206. https://doi.org/10.1007/978-3-319-93372-6_22
Kamilaris, A., & Prenafeta-Boldú, F. X. (2018). Deep learning in agriculture: A survey. Computers and Electronics in Agriculture, 147(July 2017), 70–90. https://doi.org/10.1016/j.compag.2018.02.016
Mahua, M. S. (2018). SISTEM PAKAR UNTUK MENDIAGNOSIS PENYAKIT TANAMAN JERUK ( LIMAU ) MENGGUNAKAN METODE BAYES. JATI (Jurnal Mahasiswa Teknik Informatika), 2(2), 196–202.
Mustaqim, K. (2013). Aplikasi Sistem Pakar Untuk Diagnosa Hama dan Penyakit Tanaman Kelapa Sawit Menggunakan Naive Bayes( STUDY KASUS : PT . Perkebunan Nusantara V ).
Nurhatika, S. (2013). Sistem Pakar Untuk Mendiagnosis Penyakit Tanaman Kelapa Sawit.
Ranjan, R., Patel, V. M., & Chellappa, R. (2015). A deep pyramid Deformable Part Model for face detection. 2015 IEEE 7th International Conference on Biometrics Theory, Applications and Systems, BTAS 2015. https://doi.org/10.1109/BTAS.2015.7358755
Rasywir, E., & Purwarianti, A. (2015). Eksperimen pada Sistem Klasifikasi Berita Hoax Berbahasa Indonesia Berbasis Pembelajaran Mesin. Jurnal Cybermatika, 3(2), 1–8. Retrieved from http://cybermatika.stei.itb.ac.id/ojs/index.php/cybermatika/article/view/133
Sarno, R., & Sidabutar, J. (2015). Comparison of Different Neural Network Architectures for Software Cost Estimation. In International Conference on Computer, Control, Informatics and Its Applications Comparison (pp. 68–73).
Shen, S., Bui, A. A. T., Cong, J., & Hsu, W. (2015). An automated lung segmentation approach using bidirectional chain codes to improve nodule detection accuracy. Computers in Biology and Medicine, 57, 139–149. https://doi.org/10.1016/j.compbiomed.2014.12.008
Sidauruk, A., & Pujianto, A. (2017). Sistem Pakar Diagnosa Penyakit Tanaman Kelapa Sawit menggunakan Teorema Bayes. Jurnal Ilmiah Data Manajemen Dan Teknologi Informasi, 18(maret).
Sun, C., Gan, C., & Nevatia, R. (2015). Automatic concept discovery from parallel text and visual corpora. Proceedings of the IEEE International Conference on Computer Vision, 2015 Inter, 2596–2604. https://doi.org/10.1109/ICCV.2015.298
Zhang, Y., & Mu, Z. (2017). Ear detection under uncontrolled conditions with multiple scale faster Region-based convolutional neural networks. Symmetry, 9(4). https://doi.org/10.3390/sym9040053
DOI: https://doi.org/10.31294/p.v22i2.8907
Copyright (c) 2020 Errissya Rasywir, Rudolf Sinaga, Yovi Pratama

This work is licensed under a Creative Commons Attribution-ShareAlike 4.0 International License.
ISSN: 2579-3500
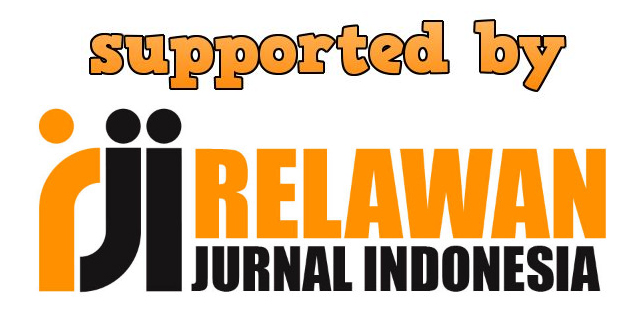
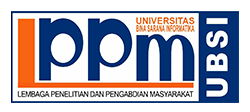