APLIKASI ALGORITMA K-MEANS UNTUK PEMETAAN MINAT NASABAH TERHADAP PRODUK ASURANSI JIWA SYARIAH
Abstract
Potential customer is someone who became accustomed to buying that formed through the changes and interactions that often during a certain period, by agreement between the seller and buyer. Mapping of potential customers by marketing analysts insurance is less accurate and difficult when the data storage media owned by large and multi dimensional. These problems required the mapping model that canclassify potential customers against certain insurance products. Model K-means algorithm can be used to mapping or classify customers based on profiles that have the potential to be an individual life insurance products with a level of accuracy reached 30%. Measurement similarity level, homogeneity and errors that are used in this study is a method of measuring cohesion and variations. Measurement method with a internal measurement methods with the Sum of Square Error.
Full Text:
PDFReferences
Berkhin, P. (2003). Survey of Clustering Data Mining Techniques. Accrue Software , 13.
Bi, J. (2010). Research for Customer Segmentation of Medical Insurance Based on K-means and C&R Tree Algorithms. 2010 Sixth International Conference on Simantics, Knowledge and Grids .
Guo, L. (2003). Applying Data Mining Techniques in Property/Casualty Insurance. Forums of the Casualty Actuarial Society.
Han, J., & Kamber, M. (2006). Data Mining: Concepts and Techniques. Morgan Kaufmann.
Hsieh, N.-C. (2004). An integrated data mining and behavioral scoring model for analyzing bank customers. Expert Systems with Applications, Elsevier Ltd. , 623-633.
Joao M. Sousa, U. K. (2002). A Comparative Study of Fuzzy Target Selection Methods in Direct Marketing. Fuzzy systems.
Kantardzic, M. (2011). Data Mining: Concepts, Models, Methods and Algorithms, Second Edition. Hoboken, NJ, USA: John Wiley & Sons, Inc.
Kanungo, T., Mount, M. D., Netanyahu, S. N., Piatko, D. C., Silverman, R., & Wu, Y. A. (2002). An Efficient k-Means Algorithm: Analysis and Implementation. IEEE Transaction on Patern Analysis and Machine Intellegence .
Larose, D. T. (2006). Data Mining methods and Models. New Jersey: Jon Wiley & Sons, Inc.
Maimon, O., & Rokach, L. (2005). Data Maining and Knowledge Discovery Handbook. New York: Springer.
RI, P., & DPR. (1992). Depkumham. Dipetik September 3, 2010, dari Media Informasi Hukum dan Peraturan Perundang-Undangan: http://www.djpp.depkumham.go.id
Sivanandam, S. (2006). Introduction to Data Mining and its Applications. Heidelberg, Berlin: Springer-Verlag.
Sugiyono. (2010). Metode Penelitian Kuantitatif kualitatif dan R&D. Bandung: Alfabeta.
Tan, P.-N., Steinbach, M., & Kumar, V. (2006). Introduction To Data Mining. Pearson Addison-Wesley.
Witten, I. H., & Frank, I. (2005). Data Mining Practical Machine Learning Tools and Techniques, Second Edition. San Francisco: Morgan Kaufmann Publishers.
DOI: https://doi.org/10.31294/p.v16i1.730
Copyright (c) 2016 . Sismadi

This work is licensed under a Creative Commons Attribution-ShareAlike 4.0 International License.
ISSN: 2579-3500
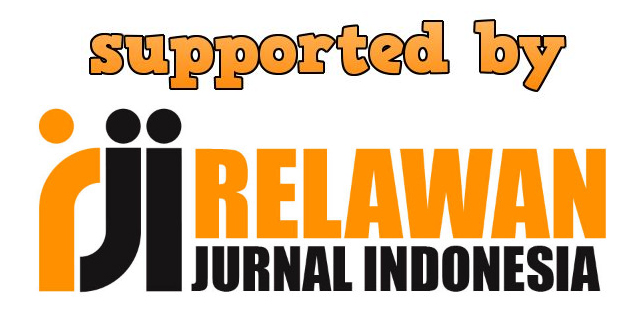
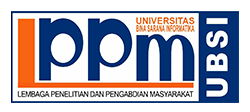