Model Prediksi Penjualan Jenis Produk Tekstil Menggunakan Algoritma K-Nearest Neighbor (K-NN)
Sari
Indonesia is a country that has a large textile industry, ranging from the synthetic fiber manufacturing industry (fiber making), the yarn spinning industry (spinning), the weaving industry (weaving), knitting industry (knitting), dyeing, printing and refinement industries to the clothing industry. so (garments). In the world of textiles, we need to pay attention to the market for the types of fabrics that will be widely produced to the public. However, there is no market survey process at this company which is caused by a lack of employees so that the company only produces goods depending on orders from customers. Therefore, it is necessary to monitor demand by customers using data mining classification so that companies can determine which types of products will be in high demand by processing the company's sales data. This study aims to assist the company in determining the direction for production and sales in the future. In this study, the algorithm chosen is data mining classification with K-Nearest Neighbor by clustering data from values k=1 to k=5. The results obtained with a value of k = 1 get an accuracy of 86.9% with an RMSE of 0.362. And the value of class recall & class precision in the confusion matrix is quite good so that the algorithm model used in this study works well.
Teks Lengkap:
PDFReferensi
Alfani, A., Rozi, F., & Sukmana, F. (2021). PREDIKSI PENJUALAN PRODUK UNILEVER MENGGUNAKAN METODE K-NEAREST NEIGHBOR. JIPI (Jurnal Ilmiah Penelitian Dan Pembelajaran Informatika).
Amalia Rizki, Y. (2018). PENERAPAN DATA MINING UNTUK PREDIKSI PENJUALAN PRODUK ELEKTRONIK TERLARIS MENGGUNAKAN METODE K-NEAREST NEIGHBOR (Studi Kasus : PT. Bintang Multi Sarana Palembang). Retrieved from http://eprints.radenfatah.ac.id/3302/
Annur, H. (2018). KLASIFIKASI MASYARAKAT MISKIN MENGGUNAKAN METODE NAÏVE BAYES. ILKOM Jurnal Ilmiah, 10, 6. Retrieved from http://103.226.139.203/index.php/ILKOM/article/view/303
Bode, A. (2017). K-NEAREST NEIGHBOR DENGAN FEATURE SELECTION MENGGUNAKAN BACKWARD ELIMINATION UNTUK PREDIKSI HARGA KOMODITI KOPI ARABIKA. ILKOM Jurnal Ilmiah, 9(2), 188–195. https://doi.org/10.33096/ilkom.v9i2.139.188-195
Cholil, S., Handayani, T., & Tria, A. (2021). Implementasi Algoritma Klasifikasi K-Nearest Neighbor (KNN) Untuk Klasifikasi Seleksi Penerima Beasiswa. IJCIT (Indonesian Journal on Computer and Information Technology), 6(2), 118–127.
Hakim, L. A. R., Rizal, A. A., & Ratnasari, D. (2019). Aplikasi Prediksi Kelulusan Mahasiswa Berbasis K-Nearest Neighbor (K-NN). JTIM : Jurnal Teknologi Informasi Dan Multimedia, 1(1), 30–36. https://doi.org/10.35746/jtim.v1i1.11
Harun, R., Pelangi, K., & Yuliyanti, L. (2020). PENERAPAN DATA MINING UNTUK MENENTUKAN POTENSI HUJAN HARIAN DENGAN MENGGUNAKAN ALGORITMA K NEAREST NEIGHBOR (KNN). MISI (Jurnal Manajemen Informatika & Sistem Informasi). Retrieved from http://e-journal.stmiklombok.ac.id/index.php/misi/article/view/125/84
Hermawan, F., & Agung, H. (2017). Implementasi Metode K-Nearest Neighbor Pada Aplikasi Data Penjualan PT. Multitek Mitra Sejati.
Wahyuningsih, S., & Utari, D. R. (2018). Perbandingan Metode K-Nearest Neighbor , Naive Bayes dan Decision Tree untuk Prediksi Kelayakan Pemberian Kredit. Konferensi Nasional Sistem Informasi 2018 STMIK Atma Luhur Pangkalpinang, 8 – 9 Maret 2018, 619–623.
Yunita. (2017). SELEKSI FITUR MENGGUNAKAN BACKWARD ELIMINATION PADA PREDIKSI CUACA. IJCIT (Indonesian Journal on Computer and Information Technology), 2(1), 26–37.
DOI: https://doi.org/10.31294/ijcit.v7i1.11973
##submission.copyrightStatement##
##submission.license.cc.by-sa4.footer##
P-ISSN: 2527-449X E-ISSN: 2549-7421
Statistik Pengunjung Jurnal IJCIT
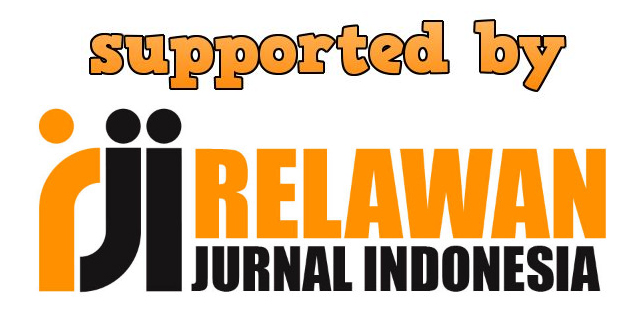
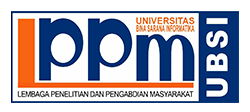