ANALISIS SENTIMEN APLIKASI TRANSPORTASI ONLINE KRL ACCESS MENGGUNAKAN METODE NAIVE BAYES
Abstract
Kata Kunci: Analisis Sentimen, Aplikasi Transportasi, Naive Bayes classifier algorithm (NBC).
ABSTRACTPublic transport is considered as a solution to problems related to urban mobility. Associated with the transport, jabodetabek have problems like other big cities in the world, traffic congestion. Traffic congestion occurs at peak hours in the morning and evening, for it needed an application that can be used to access transport easily and quickly one of which is transport applications online that is currently widely used by mobile smartphone. Sentiment analysis can be considered as a classification process that has three major classification level is the level documents, at the sentence level and at the level of aspects. At the level of sentiment analysis document aims to classify a document as a statement of opinion or the opinion of the positive and negative sentiment. In addition the use of method used in the present study was methods that are widely used in data processing in the form of text mining with a fairly high degree of accuracy that the algorithm Naive Bayes Classifier (NBC). while The Naive Bayes classifier algorithm ( NBC ) is obtained by 84.00%. Key Words: sentiment analysis,Transportation applications, Naive Bayes classifier algorithm (NBC)
Full Text:
PDF (Bahasa Indonesia)References
A.D. Reid, K. Flautner, E. Grimley-Evans, Y. Lin, SoC-C: efficient programming abstractions for heterogeneous multicore systems on chip, in: E.R. Altman (Ed.), (2008). Proceedings of the 2008 International Conference on Compilers, Architecture, and Synthesis for Embedded Systems, CASES’08, ACM, Atlanta, GA, USA, pp. 95–104.
Chou, J.-S., Cheng, M.-Y., Wu, Y.-W., & Pham, A.-D. (2014). Optimizing parameters of support vector machine using fast messy genetic algorithm for dispute classification. Expert Systems with Applications, 41(8), 3955–3964. doi:10.1016/j.eswa.2013.12.035
Hamzah, A. (2012). Klasifikasi Teks dengan Naive Bayes Classifier (NBC) untuk Pengelompokan Teks Berita dan Abstrak Akademis. Prosiding Seminar Nasional Aplikasi Sains & Teknologi (SNAST) Periode III, Yogyakarta, 269-277.
H. Guihot, Renderscript, (2012). in: Pro Android Apps Performance Optimization, Springer, pp. 231–263.
http://www. suaramerdeka.com/harian/0403/29/kot2.htm
JICA Study Team. (2004). The Study on Integrated Transportasi Master Plan for Jabodetabek (Phase II), Find Report, Main Report, I:Masterplan Study, in Coordination with National Development Planning Agency (BAPPENAS) Republic Indonesia
Manning, C. D., Ragnavan, P., & Schutze, H. (2008). An Introduction to Information Retreival. Cambridge: Cambridge University Press.
Medhat, W., Hassan, A., & Korashy, H. (2014). Sentiment analysis algorithms and applications: A survey. Ain Shams Engineering Journal doi:10.1016/j.asej.2014.04.011
Mishra, S., Welch, T.F., Jha, M.K., (2012). Performance indicators for public transit connectivity in multi-modal transportation networks. Transport. Res. Part A: Policy Practice 46 (7), 1066–1085.
Tan, P. N., Steinbach, M. & Kumar, V. (2006). Introduction to Data Mining. Boston : Pearson Addison Wesley.
Turban, E.; et.al. (2011). Decision Support and Business Intelligence Systems (9 ed.). New Jersey: Pearson Education, Inc.
Wibisono, Y. 2005. Klasifikasi Berita Berbahasa Indonesia menggunakan Naïve Bayes Classifier. [Online]. Tersedia di: http://fpmipa.upi.edu/staff/yudi/yudi_0805.pdf [diunduh: 1 Nov 2010].
DOI: https://doi.org/10.31294/swabumi.v7i1.5575
INDEXING
P-ISSN : 2355-990X E-ISSN: 2549-5178
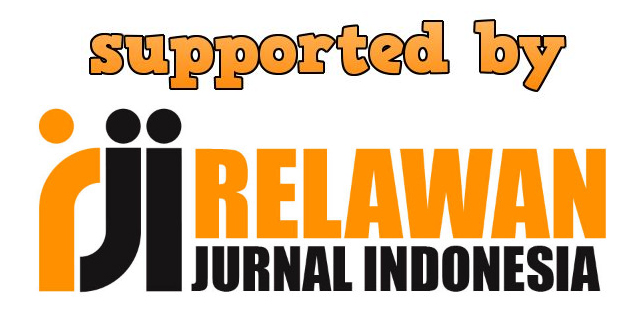
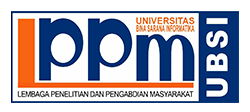