KOMPARASI ALGORITMA C4.5 DAN SVM BERBASIS PARTICLE SWARM OPTIMAZATION DALAM PENENTUAN KREDIT
Abstract
Bad credit is one of the credit risk faced by the financial and banking industry. Bad credit happens if in the long run, financial institutions or banks can not attract loans within a predetermined time. Bad credit has a negative effect on credit providers in the form of risk of loss course this should not be allowed to drag on and had to find a way out. However, to ensure accuracy in the determination of credit worthiness required an accurate algorithm. Therefore, there should be a study that aims to find an algorithm that accurately by means mengkomparasi some of them C4.5 algorithm, and SVM. To further improve the accuracy of the algorithms are in Particle Swarm Optimization with Optimazation. Berupan confusion matrix research results prove the accuracy of Support Vector Machine-based Particle Swarm Optimazation exists at the level of accuracy of 96.20% and the AUC by 0989.
Full Text:
PDFReferences
Abraham, A., Grosan, C., Ramos, V., (2006). Swarm Intelligence in Data Mining. Springer-Verlag Berlin Heidelberg.
Aydin, I., Karakose, M., & Akin, E. (2011). A multi-objective artificial immune algorithm for parameter optimization in support vector machine. Journal Applied Soft Computing, 11, 120-129.
Bellotti, T., & Crook, J. (2007) Support vector machines for credit scoring and discovery of significant features. Expert System with Application: An International Journal, 36, 3302-3308.
Edward Burst. (2006). Credit Management Handbook. USA: Gower Publishing Company.
Gorunescu, Florin (2011). Data Mining: Concepts, Models, and Techniques. Verlag Berlin Heidelberg: Springer.
Han, J., & Kamber, M. (2007). Data Mining Concepts and Technique. Morgan Kaufmann publisher.
Hian, C.K., Wei, C.T., & Chwee, P.G (2006). A Two-step Method to Construct Credit Scoring Models with Data Mining Techniques. International Journal of Business and Information, 1, 96-118.
Jianguo, Z., & Tao, B. (2008). Credit Risk Assessment using Rough Set Theory and GA-based SVM. The 3rd International Conference on Grid and Pervasive Computing, 320-325.
Larose, D. T. (2005).Discovering Knowledge in Data. New Jersey: John Willey & Sons, Inc.
Maimon, O., & Rokach, L. (2010). Data Mining and Knowledge DiscoveryHandbook. London: Springer.
Ning Liu, En Jun Xia, & Li. (2010). Research and Application of PSO-BP Neural Network in Credit Risk Assessment. International Symposium on Computational Intelligence and Design, 103-106.
Olson, D, & Shi, Y. (2008). Pengantar Ilmu Penggalian Data Bisnis. Jakarta: Penerbit Salemba Empat.
Shuzhou, W., & Bo, M. (2011). Parameter Selection Algorithm for Support Vector Machine. Procedia Environmental Sciences, 11, 538-544.
UU Perbankan No.10 Tahun 1998.
Witten, I. H., Frank, E., & Hall, M. A. (2011).Data Mining: Practical Machine Learning and Tools. Burlington: Morgan Kaufmann Publisher.
Yi Jiang, Yan Chen, Zhiming Zeng, & Xiangjian He. (2009). A Bank Customer Credit Evaluation Based on the Decision Tree and the Simulated Annealing Algorithm. World Congress on Computer Science and Information Engineering,18-22.
Yun, L., Qiu-yan, C. & Hua, Z. (2011). Application of the PSO-SVM model for Credit Scoring. Seventh International Conference on Computational Intelligence and Security, 47-51.
DOI: https://doi.org/10.31294/p.v18i1.877
Copyright (c) 2016 Syaifur Rahmatullah

This work is licensed under a Creative Commons Attribution-ShareAlike 4.0 International License.
ISSN: 2579-3500
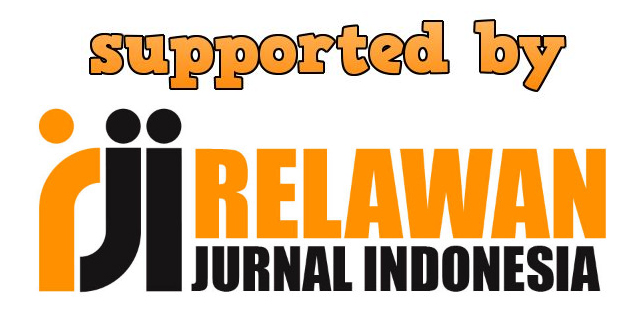
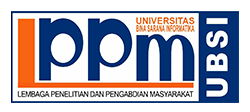