PENINGKATAN BACKWARD ELIMINATION DENGAN WINDOWED MOMENTUM UNTUK PREDIKSI KONTRASEPSI
Abstract
Rapid population growth rate that can influence government policies on various aspects of life. It is necessary for the proper way to reduce the rate of population growth and create a safer contraceptive choice. Windowed momentum is a technique to improve the performance in backpropagation learning. But to ensure the accuracy of the momentum needed windowed performance computing methods such as neural networks to solve problems with the accuracy of data and not linear. Neural Network Optimization tested weeks to produce the best accuracy rate, applying Neural Network-based Backward Elimination aims to raise the accuracy produced by Neural Network. Experiments were performed to obtain the optimal architecture and generate increased accuracy. The results of the research is a confusion matrix to prove the accuracy of Neural Network before Backward Elimination is optimized by 54.64% and 57.03% after optimize. This proves estimate windowed momentum trials using neural network-based method Backward Elimination more accurate than the individual methods of neural network.
Full Text:
PDFReferences
Alpaydin, Ethem. (2010). Introduction to Machine Learning. The MIT Press, London UK.
Alrawashdeh, Thamer A, & Muhairat, Mohammad, Ahmad Althunibat,. (2013). Evaluating the Quality of Software in ERP. Department of software Engineering, Alzaytoonah University of Jordan, Amman, Jordan.
Asliyan, Rifat. (2011). Syllable Based Speech Recognition. Computer and Information Science. Diambil dari: http://www.intechopen.com/books/speech-technologies/syllable-based-speech-recognition. (3 Desember 2014).
Bandyopadhyay, G & Chattopadhyay. (2008). An Artificial Neural Net Approach to Forecast The Population of India. India.
BKKBN. Nd. Cara-Cara Kontrasepsi yang Digunakan Dewasa Ini. Diambil dari: http://www.bkkbn-jatim.go.id/bkkbn-jatim/html/cara.htm. (3 Desember 2014).
Badan Pusat Statistik. nd. Laju Pertumbuhan Penduduk Menurut Provinsi. Diambil dari: http://bps.go.id/tab_sub/view.php?tabel=1&daftar=1&id_subyek=12¬ab=2. (3 November 2014).
Chaurasia, Aalok Ranjan. (2014). Contraceptive Use In India: A Data Mining Approach. Shyam Institute, India.
Ciampi, Antonio. Zhang, Fulin. (2002). A New Approach to Training Backpropagation Artificial Neural Network: Empirical Evaluation on Tens Dataset on Clinical Studies. McGill University. Canada.
Gorunescu, Florin. (2011). Data Mining: Concepts, Models and Techniques. Verlag Berlin Heidelberg, Springer. Jerman.
Guillet, Fabrice. Hamilton, Howard J. (2007). Quality Measures in Data Mining. Verlag Berlin Heidelberg, Springer. Jerman.
Han,J & Kamber, Micheline. (2007). Data Mining Concepts, Models and Techniques. Second Edition, Morgan Kaufmann Publisher. Elsevier.
Hagiwara, Masafumi.(1992). Theoretical Derivation of Momentum Term in Back-Propagation. International Joint Conference on Neural Networks. IEEE. pp682-686.
Heaton, Jeff. (2010). Programming Neural Networks With Encog 2 In Java. Heaton Research.Inc, USA.
Hong, X., Harris, C., Brown, M., & Chen, S. (2002). Backward Elimination Methods for Associative Memory Network Pruning. Computers and Technology, (Reed 1993).
Istook, Ernez & Martinez, Tony (2002). Improved backpropagation learning in neural networks with windowed momentum. Computer Science Department. Brigham Young University, pp. 303-318.
Kadhim, Jehan & Abdulrazzaq, Mohammad (2015). Forecasting USD/IQD Future Values According to Minimum RMSE Rate. Thi_Qar University. pg.271–285
Kohavi, R., & John, H. (1997). Artificial Intelligence Wrappers for feature subset selection. elsevier, 97(97), 273–324.
Kusumadewi, Sri & Hartati, Sri. (2010). Neuro-Fuzzy Integrasi Sistem Fuzzy & Jaringan Syaraf. Second Edition. Yogyakarta: Graha Ilmu.
Larose, D. (2005). Discovering Knowledge in Data. New Jersey, John Willey & Sons.Inc.
Liao, Warren. T. & Triantaphyllou. Evangelos. (2007). Recent Advances in Data Mining of Enterprise Data: Algorithms and Applications. Series: Computer and Operation Research. 6. 190.
Lim TS, Loh WY, Shih YS. (1999). A comparison of prediction accuracy, complexity, and training time of thirty-three old and new classification algorithms. Kluwer Academic Publishers: Boston.
Liu, Huan, Yu, Lei.(2005). Toward Integrating Feature Selection Algorithms for Classification and Clustering. Department of Computer Science and Engineering. Arizona State University.
Liu, Yuaning, Wang G., Chen, M., Dong, M., Zhu, X., Wang, S. (2011). An Improved Particle Swarm Optimization for Feature Selection. College of Computer Science and Technology. China.
Maimon, Oded & Rokach, Lior. (2010). Data Mining and Knowledge Discovery Handbook, Springer, New York.
Myatt, Glenn J. (2007). Making sense of data : A Practical Guide to Exploratory data analysis and Data Mining. John Wiley & Sons Inc, New Jersey.
Shukla, Anupam. Tiwari, Ritu. & Kala, Rahul. (2010). Real Life Application of Soft Computing. New York: Taylor and Francis Groups, LLC.
Siang, Jong Jek (2009). Jaringan Syaraf Tiruan dan Pemrogramannya menggunakan MATLAB. Penerbit Andi. Yogjakarta.
Vercellis,C. (2009). Business Intelligence: Data Mining and Optimization for Decision Making. Wiley.
Witten,I. Frank, E., & Hall. (2011). Data Mining: Practical Machine Learning and tools. Morgan Kaufmann Publisher, Burlington.
DOI: https://doi.org/10.31294/p.v17i2.749
Copyright (c) 2016 EVY PRIYANTI

This work is licensed under a Creative Commons Attribution-ShareAlike 4.0 International License.
ISSN: 2579-3500
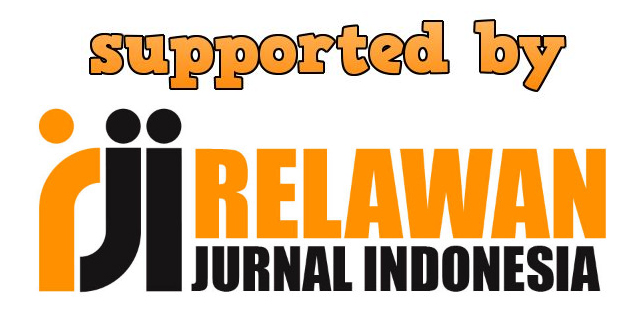
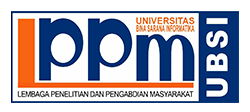