Aplikasi Diagnosa Penyakit Tuberculosis Menggunakan Algoritma Data Mining
Abstract
Full Text:
PDF (Bahasa Indonesia)References
Amrin, A. (2016). Data Mining Dengan Regresi Linier Berganda Untuk Peramalan Tingkat Inflasi. Jurnal Techno Nusa Mandiri, XIII(1), 74–79. Retrieved from http://ejournal.nusamandiri.ac.id/ejurnal/index.php/techno/article/view/268
Fine, J. (2012). An Overview Of Statistical Methods in Diagnostic Medicine. Chapel Hill.
Gorunescu, F. (2011). Data Mining: Concepts, Models, and Techniques. Verlag Berlin Heidelberg: Springer.
Han, J., & Kamber, M. (2006). Data Mining: Concepts and Techniques. Soft Computing (Vol. 54). San Fransisco: Morgan Kauffman. https://doi.org/10.1007/978-3-642-19721-5
Khotari. (2004). Data Mining Concepts and Technique. San Fransisco: Morgan Kauffman.
Kusrini, & Luthfi, E. . (2009). Algoritma Data Mining. Yogyakarta: Andi Publishing.
Liao, T. W. (2007). Recent Advances in Data Mining of Enterprise Data: Algorithms and Application. Singapore: World Scientific Publishing.
Maimon, O., & Rokach, L. (2010). Data Mining And Knowledge Discovery Handbook. New York: Springer.
Myatt, G. J. (2007). Making Sense of Data: A Practical Guide to Exploratory Data Analysis and Data Mining. New Jersey: John Wiley & Sons, Inc.
Santosa, B. (2007). Data Mining Teknik Pemanfaatan Data Untuk Keperluan Bisnis. Yogyakarta: Graha Ilmu.
Sogala, S. S. (2006). Comparing the Efficacy of the Decision Trees with Logistic Regression for Credit Risk Analysis. India.
Sumathi, S., & Sivanandam, S. N. (2006). Introduction to Data Mining and its Applications. Berlin Heidelberg New York: Springer.
Vercellis, C. (2009). Business Intelligent: Data Mining and Optimization for Decision Making. Southern Gate, Chichester, West Sussex: John Willey & Sons, Ltd.
Witten, I. H., Frank, E., & Hall, M. A. (2011). Data Mining: Practical Machine Learning and Tools. Burlington: Morgan Kaufmann.
DOI: https://doi.org/10.31294/p.v20i2.3932
Copyright (c) 2018 Amrin Amrin, Hafdiarsya Saiyar

This work is licensed under a Creative Commons Attribution-ShareAlike 4.0 International License.
ISSN: 2579-3500
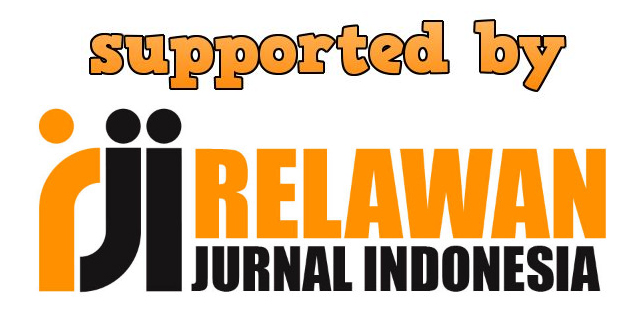
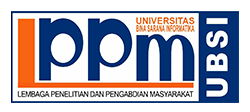