K-NEARST NEIGBOUR (KNN) UNTUK MENDETEKSI GANGGUAN JARINGAN KOMPUTER PADA INTRUSION DETECTION DATASET
Sari
Internet increasing is also exponentially increasing intrusion or attacks by crackers exploit vulnerabilitiesin Internet protocols, operating systems and software applications. Intrusion or attacks against computernet works, especially the Internet has increased from year to year. Intrusion detection systems into the main stream in the information security. The main purpose of intrusion detection system is a computer system to help deal with the attack. This study presents k-nearest neigbour algorithm to detect computer network intrusions. Performance is measured based on the level of accuracy, sensitivity, precision and spesificity. Dataset used in this study is a dataset KDD99 intrusion detection system. Dataset is composed of two training data and testing data. From the experimental results obtained by the accuracy of k-nearest neigbour algoritm is about 79,36%.
Keyword: k-nearest neigbour, intrusion detection
Teks Lengkap:
PDF (English)Referensi
Gostev, A., & Namestnikov, Y. (2011, February 17). Kaspersky Security Bulletin 2010. Statistics, 2010. Retrieved Juni 6, 2013, from Securelist: http://www.securelist.com/en/analysis/204792162/Kaspersky_Security_Bulletin_2010_Statistics_2010
Neethu, B. (2012). Classification of Intrusion Detection Dataset Using Machine Learning Approaches. International Journal of Electronics and Computer Science Engineering , 1044-1051.
Olusola, A. A., Oladele, A. S., & Abosede, D. O. (2010). Analysis of KDD '99 Intrusion Detection Dataset for Selection of Relevance Feature . World Congress on Engineering and Computer Science 2010 . San Fransisco: WCECS 2010.
Pedrycz, W., & Vukovich, G. (2001). Feature Analysis Through Information Granulation and Fuzzy Sets. (G. V. Witold Pedrycz, Ed.) Pattern Recognition, 35, 825-834.
Scarfone, K., & Mell, P. (Februari, 2007). Special Publication 800-94: Guide To Intrusion Detection and Prevention Systems . Gaithersburg, Maryland: National Institute Standard and Technology.
Tavallaee, M., Bagheri, E., Lu, W., & Ghorbani, A. A. (2009). A Detailed Analysis Of The KDD Cup 99 Data Set. Proceedings Of The 2009 IEEE Symposium On Computational Inteligence in Security and Defense Application (CISDA) (pp. 53-58). Otawa: IEEE Press Piscataway, NJ, USA.
Yu, L., & Huan, L. (2003). Feature Selection for High Dimentional Data: A Fast Correlation-Based Filter Solution. Proceeding of the Twentieth International Conference on Machine Learning (ICML-2003). Washington DC.
DOI: https://doi.org/10.31294/jki.v2i1.1624
DOI (PDF (English)): https://doi.org/10.31294/jki.v2i1.1624.g1181
##submission.copyrightStatement##
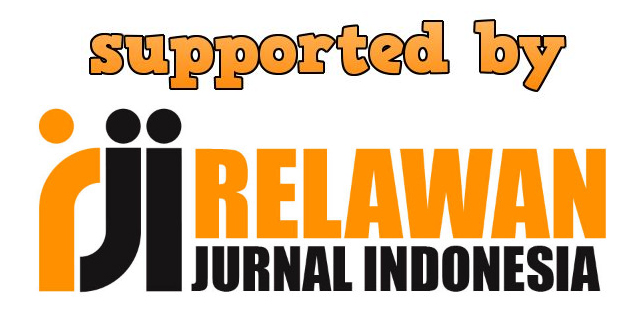
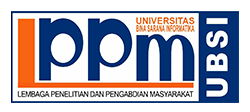
Dipublikasikan oleh LPPM Universitas Bina Sarana Informatika
Email : jurnalkhatulistiwainformatika@bsi.ac.id

This work is licensed under a Creative Commons Attribution-ShareAlike 4.0 International License