KAJIAN PENERAPAN METODE KLASIFIKASI DATA MINING ALGORITMA C4.5 UNTUK PREDIKSI KELAYAKAN KREDIT PADA BANK MAYAPADA JAKARTA
Abstract
in terms of volume of business , mobilize public funds or credit .
Data mining of the loan has great potential to explore the hidden
patterns within a dataset of loans including loans domain . C4.5
classification algorithm is the most simple , easy diimplemntasikan
. However, the algorithm C4.5 still have weaknesses in handling
high-dimensional data in . This research aims to implement the
algorithm C4.5 with the selection of attributes so as to reduce the
dimensionality of the data , and identify features in the data set
with C4.5 algorithm method . From this research, conducted
models created with C4.5 algorithm itself already has good
accuracy that is equal to 83.67 % with the selection process by the
algorithm C4.5 attributes .
Intisari — Industri perbankan mengalami perkembangan yang
cukup pesat, baik dari sisi volume usaha, mobilisasi dana
masyarakat maupun pemberian kredit. Data mining mengenai
pinjaman memiliki potensial besar untuk menjelajahi bagian
pola yang tersembunyi dalam suatu dataset dari domain
pinjaman termasuk pinjaman kredit. Algoritma C4.5
merupakan pengklasifikasian yang paling sederhana, mudah
diimplemntasikan. Namun, Algoritma C4.5 masih memiliki
kelemahan dalam menangani data dalam dimensi tinggi.
Penelitian ini bertujuan untuk menerapkan algoritma C4.5
dengan seleksi atribut sehingga dapat mengurangi dimensi dari
data, serta mengidentifikasi fitur dalam kumpulan data dengan
metode algoritma C4.5. Dari penelitian ini yang dilakukan model
yang terbentuk dengan algoritma C4.5 sendiri sudah memiliki
akurasi yang baik yaitu sebesar 83.67% dengan proses seleksi
atribut oleh algoritma C4.5.
Kata kunci — Data mining, Algoritma C4.5, kelayakan
kredit,Decision Tree
Full Text:
PDFReferences
REFERENSI
Anbarasi, M., Anupriya, E., & Iyengar, N. Enchaced 5370-Prediction of
Heart Disease with Feature Subset Selection using Genetic Algorithm.
International Journal of engineering Science and Technology , 5370-
2010.
Gorunescu, Florin. Data Mining: Concepts, Models, and Techniques.
Verlag Berlin Heidelberg: Springer . 2011.
Han, J., & Kamber, M. Data Mining Concept and Tehniques. San
Fransisco: Morgan Kauffman. 2006.
Larose Larose, D. T. Discovering Knowledge in Data. New Jersey: John
Willey & Sons,Inc. 2005.
Witten, I. H., Frank, E., & Hall, M. A. Data Mining Practical Machine
Learning Tools and Techniques. USA: Morgan Kaufmann Publishers.
Wu, X., & Kumar, V. The Top Ten Algorithms in Data Mining. New
York: Chapman & Hall/CRC. 2009.
Vercellis, Carlo. Business Intelligent:Data Mining and Optimization
forDecision Making. Southern Gate,Chichester, West Sussex: John
Willey &Sons, Ltd. 2009.
Zurada, Could Decision Trees Imnprove the Classification Accuracy and
Interpretability of Loan Granting Decisions. HICSS '10 Proceedings of
the 2010 43rd Hawaii International Conference on System Sciences, (hal.
. Koloa 2010
DOI: https://doi.org/10.31294/jtk.v2i1.371
Copyright (c) 2016 Nandang Iriadi, Nia Nuraeni

This work is licensed under a Creative Commons Attribution-ShareAlike 4.0 International License.
ISSN: 2442-2436 (print), and 2550-0120
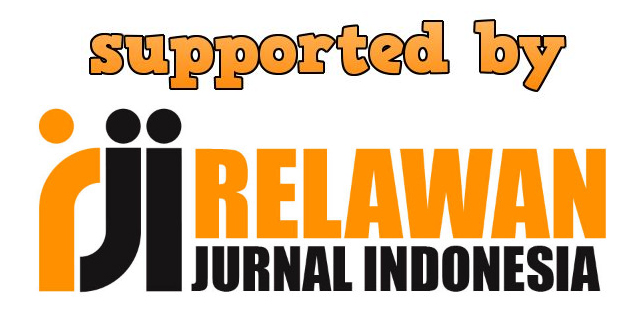
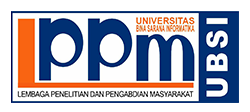