Prediksi Pemasaran Langsung Menggunakan Metode Support Vector Machine
Abstract
Abstract— Direct marketing is a typical strategy to increase business. The company uses direct marketing when targeting customer segments with their contact to fulfill a specific purpose. Direct marketing is one way that can be used to predict potential customers who open deposits at the bank. Direct marketing became a very important application in data mining today. Data mining is widely used in direct marketing to identify potential customers for new products, using the purchase history data, predictive models can be used to measure that customers will respond to a given promotion or offer. One method that is most widely used method of support vector machine. In this study will be used method of support vector machine for prediction of direct marketing. After testing the results obtained is a support vector machine produces an accuracy value of 88.71%, 89.47% and a precision value AUC value of 0.896 with a value of classification accuracy was very good (excellent clasification). Based on these results it can be concluded that the use of support vector machine method can be used for precise and accurate prediction of direct marketing.
Keywords : Prediction, Direct Marketing, Support Vector Machine.
Abstrak— Pemasaran langsung merupakan strategi yang khas untuk meningkatkan bisnis. Perusahaan menggunakan pemasaran langsung bila menargetkan segmen pelanggan dengan menghubungi mereka untuk memenuhi tujuan tertentu. pemasaran langsung merupakan salah satu cara yang dapat digunakan untuk memprediksi nasabah yang berpotensi membuka simpanan deposito pada bank tersebut. Pemasaran langsung menjadi aplikasi yang sangat penting dalam data mining saat ini. Data mining secara luas telah digunakan dalam pemasaran langsung untuk mengidentifikasi calon pelanggan untuk produk baru, dengan menggunakan data histori beli, model prediktif dapat digunakan untuk mengukur bahwa pelanggan akan menanggapi promosi atau tawaran yang diberikan. Salah satu metode yang paling banyak digunakan adalah metode support vector machine. Dalam penelitian ini akan digunakan metode support vector machine untuk prediksi pemasaran langsung. Setelah dilakukan pengujian maka hasil yang didapat adalah support vector machine menghasilkan nilai akurasi sebesar 88,71 %, nilai precision 89,47% dan nilai AUC sebesar 0,896 dengan nilai akurasi klasifikasi sangat baik (excellent clasification). Berdasarkan hasil tersebut dapat disimpulkan bahwa penggunaan metode support vector machine dapat digunakan secara tepat dan akurat untuk prediksi pemasaran langsung.
Kata Kunci— Prediksi, Pemasaran Langsung, Support Vector Machine.
Full Text:
PDFReferences
Samsu Adi Nugroho. (2016, Agustus) Lembaga Penjamin Simpanan. [Online]. http://www.lps.go.id/siaran-pers/-/asset_publisher/1T0a/content/press-release-distribusi-simpanan-bank-umum-agustus-2016
Undang-Undang Republik Indonesia Nomor 10 Tahun 1998. (2016, Agustus) Badan Pengawas Keuangan dan Pembangunan. [Online]. www.bpkp.go.id/uu/filedownload/2/45/435.bpkp
Niharika Sharma, Arvinder Kaur, Sheetal Gandotra, and Bhawna Sharma, "Evaluation and Comparison of Data Mining Techniques Over Bank Direct Marketing," IJIRSET, vol. IV, pp. 7141-7147, Agustus 2015.
Ian Witten, Eibe Frank, and Mark Hall, Data Mining Practical Machine Learning Tools and Techniques, 3rd ed., Morgan Kaufmann, Ed. United States of America: Elsevier, 2011.
S Moro and R. M. S. Laureano, "Using Data Mining for Bank Direct Marketing: An application of the CRISP-DM methodology," European Simulation and Modelling Conference, vol. Figure I, pp. 117-121, 2012.
H. A Elsalamony and A. M Elsayad, "Bank Direct Marketing Based on Neural Network and C5.0 Models," IJEAT, vol. II, no. 6, pp. 392-400, Agustus 2013.
William Chitty, Barker Nigel, and A. Shimp Terence, Integrated Marketing Communication, 2nd ed. Australia: Thomas Nelson Australia, 2008.
A Brinson, Min-Yang Lee, and Barbara Rountree, "Direct marketing strategies: The rise of community supported fishery programs," Marine Policy, pp. 542-548, Januari 2011.
F Talla, R Leus, and F. C. R. Spieksma, "Optimization models for targeted offers in direct marketing: Exact and Heuristic Algorithms," European Journal of Operational Research, pp. 670-683, Oktober 2011.
S. Liao, Y. Chen, and H. Hsieh, "Mining customer knowledge for direct selling and marketing," Expert Systems with Applications, pp. 6059-6069, 2011.
I. H Written, E. Frank, and M. A. Hall, Data Mining Practical Machine Learning and Tools. Burlington, USA: Morgan Koufmann Publisher, 2011.
Gorunescu and Florin, Data Mining: Concepts, Models, and Techniques. Verlag Berlin Heidelberg: Springer, 2011.
J. Han and M. Kamber, Data Mining Concepts and technique. San Francisco, USA: Diane Cerra, 2007.
T. Bellotti and J. Crook, "Support vector machines for credit scoring and discovery of significant features," Expert System with Application: An International Journal, no. 36, pp. 3302-3308, 2007.
I. Aydin, M. Karakose, and E. Akin, "A multi-objective artificial immune algorithm for parameter optimization in support vector machine," Journal Applied Soft Computing, no. 11, pp. 120-129, 2011.
H. Yin, Jiao X., Y. Chai, and B. Fang, "Scene classification based on single-layer SAE and SVM," Expert Systems with Applications, vol. 7, no. 42, pp. 3368-3380, 2015.
G. Li, J. You, and X. Liu, "Support Vector Machine (SVM) based prestack AVO inversion and its applications," Journal of Applied Geophysics, pp. 60-68, 2015.
S Moro and R.M.S. Laureano, "Using Data Mining for Bank Direct Marketing: An Application of the CRISP-DM methodology," European Simulation and Modelling Conference, pp. 117-121, 2012.
E. Prasetyo, Data Mining : Konsep dan Aplikasi Menggunakan Matlab. Indonesia: Andi Yogyakarta, 2012.
Pin Lio et al., "Parameter Optimization for Support Vector Machine Based on Nested Genetic Algorthms," Journal of Automation and Control Engineering, vol. 3, 2015.
Chena. Fei-Long and Feng-Chia Li., "Combination of Feature Selection Approaches with SVM in Credit Scoring," Expert Systems with Applications: An International Journal archive, vol. 37, no. 7, pp. 4902-4909, 2010.
DOI: https://doi.org/10.31294/jtk.v3i2.1719
Copyright (c) 2017 Yuni Eka Achyani

This work is licensed under a Creative Commons Attribution-ShareAlike 4.0 International License.
ISSN: 2442-2436 (print), and 2550-0120
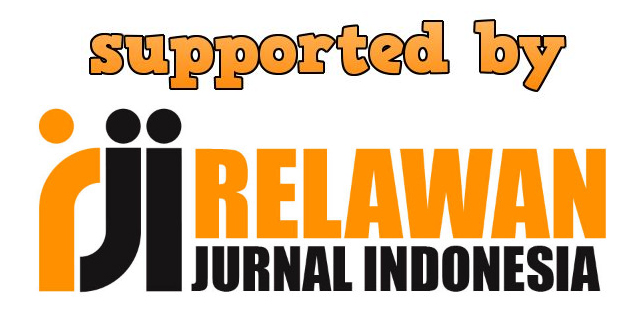
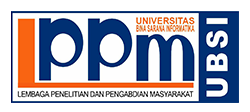