Diagnosa Tuberculosis Paru Berbasis Citra X-ray Menggunakan Convolutional Neural Network
Sari
ABSTRAK
Tuberkulosis merupakan sebuah penyakit yang disebabkan oleh Mycobacterium tuberculosis. Penyakit ini dapat menyerang darah, tulang otak dan paru-paru. Diagnosa yang cepat dan akurat sangat diperlukan agar dapat dilakukan pengobatan yang tepat. Diagnosa biasanya dilakukan dengan cara melihat hasil citra x-ray thorax dan hasil test BTA pada pasien. Penelitian ini bertujuan untuk mempercepat identifikasi dalam proses diagnosa dari citra paru yang terinfeksi bakteri tuberculosis. Diagnosa dilakukan dengan bantuan machine learning berdasarkan hasil citra x-ray menggunakan Algoritma CNN, dengan cara mengklasifikasikan citra x-ray normal dan citra x-ray tuberculosis. Dalam penelitian ini, dibahas tentang penggunaan citra x-ray paru atau citra thorax untuk mendeteksi diagnosa penyakit paru yang disebabkan oleh microbacterium tuberculosis. Hasil klasifikasi dengan CNN yang didapat setelah proses evaluasi model menunjukan nilai yang cukup baik yaitu untuk nilai akurasi di kisaran 89%, sementara untuk nilai f1-score 0,89
ABSTRACTS
Tuberculosis is a disesase caused by microbacterium tuberculosis. This disease can attack the blood, bones, barain and lungs. A fast and accurate diagnosis is nedded so that appropriate treatment can be carried out. In case tuberculosis, diagnostic is usually done by the result of the chest x-ray image, and the diagnostic result by laboratories BTA test on the patient. This research aims to accelerated the identification in the diagnostic process of lungs image infeted tuberculosis bactria diagnosis is done with the help of machine learning based on the results of x-ray images using the CNN Algorithm, by classifying normal x-ray images and tuberculosis x-ray images. In this study, we discussed the use of the thorax image for diagnostic disease lungs caused by microbacterium tuberculosis. The classification results with CNN obtained after the model evaluation process showed a fairly good value, namely for the accuracy value in the range of 89%, while for the f1-score value of 0,89.
Teks Lengkap:
PDFReferensi
Alawi, A. E. B., Al-Basser, A., Sallam, A., Al-Sabaeei, A., & Al-Khateeb, H. (2021). Convolutional Neural Networks Model for Screening Tuberculosis Disease. 2021 International Conference of Technology, Science and Administration, ICTSA 2021. https://doi.org/10.1109/ICTSA52017.2021.9406520
Higashiguchi, M., Nishioka, K., Kimura, H., & Matsumoto, T. (2021). Prediction of the duration needed to achieve culture negativity in patients with active pulmonary tuberculosis using convolutional neural networks and chest radiography. Respiratory Investigation, 59(4), 421–427. https://doi.org/10.1016/j.resinv.2021.01.004
Kementerian Kesehatan RI. (2018). Info Datin TBC. In Kemenkes RI (Vol. 67, Issue 1).
Ladumor, H., Al-Mohannadi, S., Ameerudeen, F. S., Ladumor, S., & Fadl, S. (2021). TB or not TB: A comprehensive review of imaging manifestations of abdominal tuberculosis and its mimics. Clinical Imaging, 76, 130–143. https://doi.org/10.1016/j.clinimag.2021.02.012
Lopez-Garnier, S., Sheen, P., & Zimic, M. (2019). Automatic diagnostics of tuberculosis using convolutional neural networks analysis of MODS digital images. PLoS ONE, 14(2). https://doi.org/10.1371/journal.pone.0212094
Oloko-Oba, M., & Viriri, S. (2020). Diagnosing tuberculosis using deep convolutional neural network. Lecture Notes in Computer Science (Including Subseries Lecture Notes in Artificial Intelligence and Lecture Notes in Bioinformatics), 12119 LNCS, 151–161. https://doi.org/10.1007/978-3-030-51935-3_16
Sathitratanacheewin, S., Sunanta, P., & Pongpirul, K. (2020). Deep learning for automated classification of tuberculosis-related chest X-Ray: dataset distribution shift limits diagnostic performance generalizability. Heliyon, 6(8). https://doi.org/10.1016/j.heliyon.2020.e04614
Syafiq, M., Saaidi, I., Yusoff, M., Sadikin, A., Afendi, M., & Hassan, A. M. (2021). Tuberculosis X-Ray Images Classification based Dynamic Update Particle Swarm Optimization with CNN. 48(9).
Tavolara, T. E., Niazi, M. K. K., Ginese, M., Piedra-Mora, C., Gatti, D. M., Beamer, G., & Gurcan, M. N. (2020). Automatic discovery of clinically interpretable imaging biomarkers for Mycobacterium tuberculosis supersusceptibility using deep learning. EBioMedicine, 62. https://doi.org/10.1016/j.ebiom.2020.103094
Wang, X., Peng, Y., Lu, L., Lu, Z., Bagheri, M., & Summers, R. M. (2017). ChestX-ray8: Hospital-scale chest X-ray database and benchmarks on weakly-supervised classification and localization of common thorax diseases. Proceedings - 30th IEEE Conference on Computer Vision and Pattern Recognition, CVPR 2017, 2017-Janua, 3462–3471. https://doi.org/10.1109/CVPR.2017.369
Zhong, S., Hu, J., Yu, X., & Zhang, H. (2021). Molecular image-convolutional neural network (CNN) assisted QSAR models for predicting contaminant reactivity toward OH radicals: Transfer learning, data augmentation and model interpretation. Chemical Engineering Journal, 408. https://doi.org/10.1016/j.cej.2020.127998
DOI: https://doi.org/10.31294/ijcit.v6i2.11844
##submission.copyrightStatement##
##submission.license.cc.by-sa4.footer##
P-ISSN: 2527-449X E-ISSN: 2549-7421
Statistik Pengunjung Jurnal IJCIT
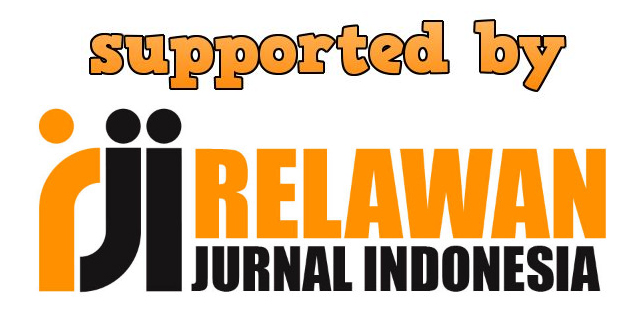
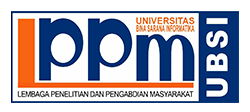