PENERAPAN PRINCIPAL COMPONENT ANALYSIS DAN GENETIC ALGORITHM PADA ANALISIS SENTIMEN REVIEW PENGIRIMAN BARANG MENGGUNAKAN ALGORITMA SUPPORT VECTOR MACHINE
Abstract
Abstract - Sentiment analysis studied the opinion of people, sentiments, attitudes, assessment, evaluation, and emotions against entities such as products, services, events, organizations, individuals, issues, topics and attributes. This information may be used for market research, product feedback and analyze the effectiveness of customer service. The problem in this research is the selection of features to improve the accuracy of Support Vector Machine and find the parameter values to get the highest accuracy in sentiment analysis review of the delivery of goods as well as produce a classification of negative and positive results of the review appropriately. Author using Principal Component Analysis and Genetic Algorithm as an optimization to improve the accuracy of Support Vector Machine method. The resulting accuracy of the algorithm Support Vector Machine by 86.00%, after optimized by using Principal Component Analysis and Genetic Algorithm accuracy has increased to 97.00%.
Keywords: Sentiment Analysis, Review of Goods Delivery, Support Vector Machine.
Abstrak - Analisis sentimen mempelajari pendapat orang, sentimen, sikap, penilaian, evaluasi, dan emosi terhadap entitas seperti produk, layanan, kejadian, organisasi, individu, isu, topik dan atribut. Informasi ini dapat digunakan untuk riset pasar, umpan balik produk dan analisis efektivitas layanan pelanggan. Masalah dalam penelitian ini adalah pemilihan fitur untuk meningkatkan akurasi Support Vector Machine dan menemukan nilai parameter untuk mendapatkan akurasi tertinggi dalam analisis sentimen tinjauan terhadap pengiriman barang serta menghasilkan klasifikasi hasil negatif dan positif dari tinjau dengan tepat Penulis menggunakan Principal Component Analysis dan Genetic Algorithm sebagai optimasi untuk meningkatkan akurasi metode Support Vector Machine. Keakuratan yang dihasilkan dari algoritma Support Vector Machine sebesar 86.00%, setelah dioptimalkan dengan menggunakan Principal Component Analysis dan Genetic Algorithm accuracy telah meningkat menjadi 97%.
Kata Kunci: Analisis Sentimen, Review Pengiriman Barang, Support Vector Machine.
Full Text:
PDFReferences
Basari, A. S. H., Hussin, B., Ananta, I. G. P., & Zeniarja, J. 2013. Opinion Mining of Movie Review using Hybrid Method of Support Vector Machine and Particle Swarm Optimization. Procedia Engineering, 453–462. http://doi.org/10.1016/j.proeng.2013.02.059
Chandani, V. 2015. Komparasi Algoritma Klasifikasi Machine Learning Dan Feature Selection pada Analisis Sentimen Review Film, Journal of Intelligent Systems, 55–59.
Dawson, C. W. 2009. Projects in Computing and Information Systems. England: Pearson Education Limited.
Elkan, C. 2013. Predictive analytics and data mining. Technical report, Cato Institute.
Feldman. 2007. The Text Mining Handbook. New York: Cambridge Univesity Press.
Ghiassi, M., Skinner, J., & Zimbra, D. 2013. Twitter brand sentiment analysis: A hybrid system using n-gram analysis and dynamic artificial neural network. Expert Systems with Applications, 40(16), 6266–6282. http://doi.org/10.1016/j.eswa.2013.05.057
Gorunescu, F. 2011. Data mining: concepts and techniques. Springer-Verlag Berlin Heidelberg. http://doi.org/10.1007/978-3-642-19721-5
Gunal. 2012. Hybrid feature selection for text classification, Turk J Elec Eng & Comp Sci, 20. http://doi.org/10.3906/elk-1101-1064
Kontopoulos, E., Berberidis, C., Dergiades, T., & Bassiliades, N. 2013. Ontology-based sentiment analysis of twitter posts. Expert Systems with Applications, 40(10), 4065–4074. http://doi.org/10.1016/j.eswa.2013.01.001
Kurniawan. 2013. Optimasi Algoritma Support Vector Machine (SVM), Jurnal Teknologi Informasi, 9 (April), 38–49.
Liu, B. 2010. Sentiment Analysis and Subjectivity, 1–38. Chicago: Handbook of Natural Language Processing
Liu, B. 2012. Sentiment Analysis and Opinion Mining. Chicago: Morgan & Claypool Publishers.
Made, N., Lestari, A., Gede, I. K., Putra, D., Ketut, A. A., & Cahyawan, A. 2013. Personality Types Classification for Indonesian Text in Partners Searching Website Using Naïve Bayes Methods, 10(1), 1–8.
Miner, G., Delen, D. 2012. The Seven Practice Areas of Text Analytics, (January), 29–41. Elsevier Inc. http://doi.org/10.1016/B978-0-12-386979-1.00002-5
Moraes, R., Valiati, J. F., & Gavião Neto, W. P. 2013. Document-level sentiment classification: An empirical comparison between SVM and ANN. Expert Systems with Applications, 40(2), 621–633. http://doi.org/10.1016/j.eswa.2012.07.059
Morchid, M., Dufour, R., Bousquet, P.-M., Linarès, G., & Torres-Moreno, J.-M. 2014. Feature selection using Principal Component Analysis for massive retweet detection. Pattern Recognition Letters, 49, 33–39. http://doi.org/10.1016/j.patrec.2014.05.020
Noviati. 2015. Analisis Pengaruh Seleksi Fitur pada Klasifikasi Konsentrasi. Semarang. Jurnal Nasional Sains dan Teknologi.
Pang, B., & Lee, L. 2008. Opinion mining and sentiment analysis. Foundations and Trends in Information Retrieval. 2 (1).
Pratama, E. E., & Trilaksono, B. R. 2014. Klasifikasi Keluhan Pelanggan Berdasarkan Tweet dengan Menggunakan Metode Support Vector Machine ( SVM ). Bandung: Jurnal Teknik Elektro dan Informatika.
Puspaningrum, E. Y., & Syidada, S. 2010. Reduksi Fitur Untuk Kategorisasi Text Dengan, 2–5. Surabaya: Jurnal Teknik Informatika.
Puspitaningrum. 2014. Dampak Reduksi Sampel Menggunakan Principal Component Analysis (PCA) Pada Pelatihan Jaringan Saraf Tiruan Terawasi - 2014. Bengkulu: Jurnal Pseudocode.
Sartono. 2010. Pengenalan Algoritma Genetik Untuk Pemilihan Peubah Penjelas Dalam Model Regresi Menggunakan SAS / IML ( An Introduction to Variables Selection in Regression Analysis using Genetic Algorithm with SAS / IML ) Bagus Sartono Peneliti di Data Mining Center – , 15(2), 10–15.
Singh, P., & Pandi, G. S. 2015. Opinion Mining Techniques for Social Network Analysis : A Survey, 2(12), 350–354. International Journal for Scientific Research & Development.
Swati. 2015. SVM Based Improvement In KNN For Text. International Journal of Engineering Research and General Science. 3(4), 214–220.
Tan, S., & Zhang, J. 2008. An empirical study of sentiment analysis for chinese documents. Expert Systems with Applications, 34(4), 2622–2629. http://doi.org/10.1016/j.eswa.2007.05.028
Thakor, P., & Sasi, S. 2015. Ontology-based Sentiment Analysis Process for Social Media Content. Procedia Computer Science, 53, 199–207. http://doi.org/10.1016/j.procs.2015.07.295
Vercellis, C. 2009. Business Intelligence: Data Mining and Optimization for Decision Making. United Kingdom: A John Wiley and Sons, Ltd., Publication.
Wahyuni, D. T., & Luthfiarta, A. 2004. Prediksi Hasil Pemilu Legislatif DKI Jakarta Menggunakan Naïve Bayes Dengan Algoritma Genetika Sebagai Fitur Seleksi. Semarang: Jurnal Teknik Informatika FIK UDINUS.
Wan, C. H., Lee, L. H., Rajkumar, R., & Isa, D. 2012. A hybrid text classification approach with low dependency on parameter by integrating K-nearest neighbor and support vector machine. Expert Systems with Applications, 39(15), 11880–11888. http://doi.org/10.1016/j.eswa.2012.02.068
Wang, Z. 2015. Enhancing Machine-Learning Methods for Sentiment Classification of Web Data Enhancing Machine-Learning Methods for Sentiment, (September). Switzerland: Springer International Publishing.
Wayan, N., Saraswati, S., Prodi, S., Informatika, T., Denpasar, S., Tukad, J., & No, B. 2013. Naïve Bayes Classifier Dan Support Vector Machines Untuk Sentiment Analysis, 2–4. Bali: Seminar Nasional Sistem Informasi Indonesia.
Zhang, Z., Ye, Q., Zhang, Z., & Li, Y. 2011. Sentiment classification of Internet restaurant reviews written in Cantonese. Expert Systems with Applications, 38(6), 7674–7682. http://doi.org/10.1016/j.eswa.2010.12.147
DOI: https://doi.org/10.31294/evolusi.v5i2.3130
ISSN: 2657-0793 (online). ISSN: 2338-8161 (print)
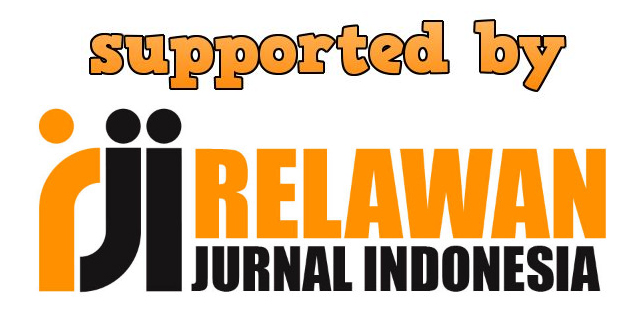
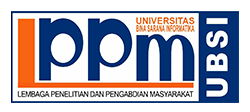