PENERAPAN PARTICLE SWARM OPTIMIZATION SEBAGAI SELEKSI FITUR PREDIKSI KELAHIRAN PREMATUR PADA ALGORITMA NEURAL NETWORK
Abstract
at a gestational age 20-36 week.The research related by birth
prematurely been carried out by researchers are using the neural
network. But the research only manyajikan about the sensitivity and
specificity. Research using methods neural network in the predicted
birth prematurely to have the kind of accuracy that results are not
enough and accurate are only serving about the sensitivity and
specificity.In this research there have been built a model algorithms
neural network and models algorithms neural network based
particle swarm optimization to get architecture in forecasting
premature birth and put a value the kind of accuracy that more
accurately at the data set patients sumber waras hospital.After
testing is conducted with two models and algorithms network neural
algorithms based particle swarm neural network optimization and
the result obtained is algorithms neural network yielding 96,40
percent of the value of accuracy and value of 0,982 but after the
auc conducted the addition of which neural algorithms based
particle swarm network optimization 96,80 percent of the value of
accuracy and value of 0,987 auc .So both have the method accuracy
of the different levels namely 0.40 percent of the auc 0,005 and the
difference .
Intisari-Persalinan prematur, didefinisikan sebagai persalinan pada
wanita hamil dengan usia kehamilan 20 – 36 minggu. Penelitian
yang berhubungan dengan kelahiran prematur sudah pernah
dilakukan oleh peneliti yaitu dengan menggunakan metode neural
network. Namun penelitian tersebut hanya manyajikan tentang
hasil sensitivitas dan spesifisitas. Hasil Penelitian yang
menggunakan metode neural network dalam memprediksi
kelahiran prematur mempunyai nilai akurasi yang dihasilkan
masih kurang akurat dan hanya sebatas menyajikan tentang hasil
sensitivitas dan spesifisitas. Dalam penelitian ini dibuatkan model
algoritma neural network dan model algoritma neural network
berbasis particle swarm optimization untuk mendapatkan arsitektur
dalam memprediksi kelahiran prematur dan memberikan nilai
akurasi yang lebih akurat pada data set pasien RS Sumber Waras.
Setelah dilakukan pengujian dengan dua model yaitu algoritma
neural network dan algoritma neural network berbasis particle
swarm optimization maka hasil yang didapat adalah algoritma
neural network menghasilkan nilai akurasi sebesar 96,40% dan
nilai AUC sebesar 0,982 namun setelah dilakukan penambahan
yaitu algoritma neural network berbasis particle swarm
optimization nilai akurasi sebesar 96,80 % dan nilai AUC sebesar
0,987.Sehingga kedua metode tersebut memiliki perbedaan tingkat
akurasi yaitu sebesar 0,40 % dan perbedaan nilai AUC sebesar
0,005.
Kata Kunci: Kelahiran prematur , Neural network , Particle
Swarm Optimization
Full Text:
PDFReferences
. Astuti, E. D. Pengantar Jaringan Saraf Tiruan. Wonosobo: Star
Publishing. 2009.
. Berndtssom, M., Hansson, J., Olsson, B., & Lundell, B. A Guide for
Students in Computer Science and Information Systems. London:
Springer. 2008.
. Catley,C.,Frize,M.,Walker,R.,Petriu,C. Predicting High-Risk
Prematur Birth Using Artificial Neural Networks. IEEE
TRANSACTIONS ON INFORMATION TECHNOLOGY IN
BIOMEDICINE, VOL. 10, NO. 3, JULY 2006.540-549. 2006.
. Dawson, C. W. Projects in Computing and Information System A
Student's Guide. England: Addison-Wesley. 2009.
. Han, J., & Kamber, M. Data Mining Concepts and Technique.
Morgan Kaufmann publisher. 2007.
. Health Technology Assessment Indonesia,. Prediksi Persalinan
Prematur. Dirjen Bina Pelayanan Medik Kemenetrian Kesehatan
Republik Indonesia. 2009.
. Ling, S. H., Nguyen, H. T., & Chan, K. Y. A New Particle Swarm
Optimization Algorithm for Neural Network Optimization. Network
and System Security, third International Conference , 516-521. 2009.
. Park, T. S., Lee, J. H., & Choi, B. Optimization for Artificial Neural
Network with Adaptive inertial weight of particle swarm
optimization. Cognitive Informatics, IEEE International Conference ,
-485. 2009.
. Salappa, A., Doumpos, M., & Zopounidis, C. Feature Selection
Algorithms in Classification Problems: An Experimental Evaluation.
Systems Analysis, Optimization and Data Mining in Biomedicine ,
-212. 2007.
. Santosa, B,. Data mining teknik pemanfaatan data untuk keperluan
bisnis. Yogyakarta: Graha ilmu. 2007.
. Shukla, A., Tiwari, R., & Kala, R. Real Life Application of Soft
Computing. CRC Press. 2010.
. Vercellis, C. Business Intelligence : Data Mining and Optimization
for Decision Making. John Wiley & Sons, Ltd. 2009.
. Witten, I. H., Frank, E., & Hall, M. A. .Data Mining: Practical
Machine Learning and Tools. Burlington: Morgan Kaufmann
Publisher. 2011.
DOI: https://doi.org/10.31294/jtk.v1i2.249
Copyright (c) 2015 Kresna Ramanda

This work is licensed under a Creative Commons Attribution-ShareAlike 4.0 International License.
ISSN: 2442-2436 (print), and 2550-0120
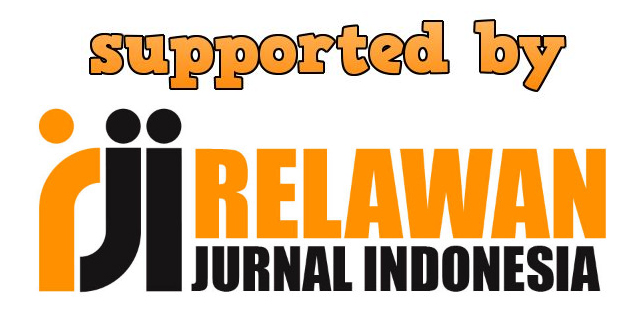
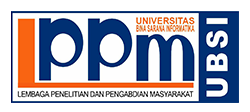