Prediksi Status Pinjaman Bank dengan Deep Learning Neural Network
Abstract
Keywords
Full Text:
PDFReferences
Addo, P. M., Guegan, D., & Hassani, B. (2018). Credit risk analysis using machine and deep learning models. Risks, 6(2), 1–20. https://doi.org/10.3390/risks6020038
Agarap, A. F. M. (2018). Deep Learning using Rectified Linear Units (ReLU). ArXiv, 1, 2–8.
Arutjothi, G. (2017). Prediction of Loan Status in Commercial Bank using Machine Learning Classifier. 2017 International Conference on Intelligent Sustainable Systems (ICISS), Iciss, 416–419.
Duan, J. (2019). Financial system modeling using deep neural networks (DNNs) for effective risk assessment and prediction. Journal of the Franklin Institute, 356(8), 4716–4731. https://doi.org/10.1016/j.jfranklin.2019.01.046
Economics, A., & Xxvi, V. (2019). A Deep Neural Network (DNN) based classification model in application to loan default prediction. Theoretical and Applied Economics, XXVI(4), 75–84.
Fan, Q., & Yang, J. (2018). A denoising autoencoder approach for credit risk analysis. ACM International Conference Proceeding Series, 62–65. https://doi.org/10.1145/3194452.3194456
Fine, T. L. (2001). Feedforward Neural Network Methodology. IEEE Transactions on Neural Networks, 12(3), 647–648. https://doi.org/10.1109/TNN.2001.925573
Golbayani, P., Wang, D., & Florescu, I. (2020). Application of Deep Neural Networks to assess corporate Credit Rating. ArXiv, 1–18.
Kanapickiene, R., & Spicas, R. (2019). Credit risk assessment model for small and micro-enterprises: The case of Lithuania. Risks, 7(2), 1–23. https://doi.org/10.3390/risks7020067
Kumar Gupta, D., & Goyal, S. (2018). Credit Risk Prediction Using Artificial Neural Network Algorithm. International Journal of Modern Education and Computer Science, 10(5), 9–16. https://doi.org/10.5815/ijmecs.2018.05.02
Li, Y., Lin, X., Wang, X., Shen, F., & Gong, Z. (2018). Credit Risk Assessment Algorithm Using Deep Neural Networks with Clustering and Merging. Proceedings - 13th International Conference on Computational Intelligence and Security, CIS 2017, 2018-Janua, 173–176. https://doi.org/10.1109/CIS.2017.00045
Lu, Y., Wang, Y., Zhang, Y., Lu, Y., Yu, X., & Wang, Y. (2020). A Comparative Assessment of Credit Risk Model Based on Machine Learning. Procedia Computer Science, 174, 141–149. https://doi.org/10.1016/j.procs.2020.06.069
Nwankpa, C. E., Ijomah, W., Gachagan, A., & Marshall, S. (2018). Activation functions: Comparison of trends in practice and research for deep learning. ArXiv, 1–20.
Patel, B., Patil, H., Hembram, J., & Jaswal, S. (2020). Loan default forecasting using data mining. 2020 International Conference for Emerging Technology, INCET 2020, 7–10. https://doi.org/10.1109/INCET49848.2020.9154100
PricewaterhouseCoopers. (2018). 2018 Indonesia Banking Survey Technology shift in Indonesia is underway. February, 48.
Suhermi, N., Suhartono, S., Dana, I. M. G. M., & Prastyo, D. D. (2019). Pemilihan Arsitektur Terbaik pada Model Deep Learning Melalui Pendekatan Desain Eksperimen untuk Peramalan Deret Waktu Nonlinier. STATISTIKA: Journal of Theoretical Statistics and Its Applications, 18(2), 153–159. https://doi.org/10.29313/jstat.v18i2.4545
Syafrudin, S., Nugraha, A. R., & Handayani, K. (2021). Prediksi Status Pinjaman Bank dengan Deep Learning Neural Network (DNN).
DOI: https://doi.org/10.31294/jtk.v7i2.10474
Copyright (c) 2021 Sukri Syafrudin, Ranu Agastya Nugraha, Kartika Handayani, Safitri Linawati, Windu Gata

This work is licensed under a Creative Commons Attribution-ShareAlike 4.0 International License.
ISSN: 2442-2436 (print), and 2550-0120
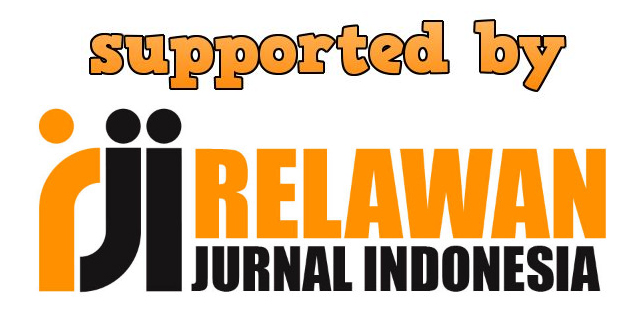
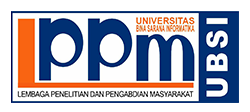