Forward Selection pada Support Vector Machine untuk Memprediksi Kanker Payudara
Sari
Kanker payudara merupakan masalah kesehatan yang serius, sehingga deteksi dini dari kanker payudara dapat berperan penting dalam perencanaan pengobatan. Pada penelitian ini Support Vector Machine dengan kernel (dot, polynomial, RBF) dan forward selection diterapkan. Perbandingan akurasi SVM tanpa forward selection dengan menggunakan forward selection menunjukkan selisih yang besar. Hasil penelitian menunjukkan SVM(RBF)+FS unggul dengan akurasi 85,38% dibandingkan dengan SVM(Polynomial & dot), selain itu SVM(RBF)+FS juga unggul dibandingkan algoritma machine learning lainnya dalam memprediksi dataset kanker payudara Coimbra.
Teks Lengkap:
PDFReferensi
Astuti, F. D. (2018). Seleksi Fitur Forward Selection pada Algoritma Naive Bayes untuk Klasifikasi Benih Gandum. Jurnal Informasi Interaktif, 3(1), 161–166.
Balram, D., Lian, K. Y., & Sebastian, N. (2019). Air quality warning system based on a localized PM2.5 soft sensor using a novel approach of Bayesian regularized neural network via forward feature selection. Ecotoxicology and Environmental Safety, 182(April), 109386.
https://doi.org/10.1016/j.ecoenv.2019.109386
Bergman, J., Schrempf, D., Kosiol, C., & Vogl, C. (2018). Inference in population genetics using forward and backward, discrete and continuous time processes. Journal of Theoretical Biology, 439, 166–180. https://doi.org/10.1016/j.jtbi.2017.12.008
Bustamam, A., Bachtiar, A., & Sarwinda, D. (2019). Selecting Features Subsets Based on Support Vector Machine-Recursive Features Elimination and One Dimensional-Naïve Bayes Classifier using Support Vector Machines for Classification of Prostate and Breast Cancer. Procedia Computer Science, 157, 450–458. https://doi.org/10.1016/j.procs.2019.08.238
Ellmann, S., Seyler, L., Evers, J., Heinen, H., Bozec, A., Prante, O., … Bäuerle, T. (2019). Prediction of early metastatic disease in experimental breast cancer bone metastasis by combining PET/CT and MRI parameters to a Model-Averaged Neural Network. Bone, 120, 254–261.https://doi.org/10.1016/j.bone.2018.11.008
Fallahpour, S., Lakvan, E. N., & Zadeh, M. H. (2017). Using an ensemble classifier based on sequential floating forward selection for financial distress prediction problem. Journal of Retailing and Consumer Services, 34(March 2016), 159–167. https://doi.org/10.1016/j.jretconser.2016.10.002
Harafani, H., & Wahono, R. S. (2015). Optimasi Parameter pada Support Vector Machine Berbasis Algoritma Genetika untuk Estimasi Kebakaran Hutan. Journal of Intelligent Systems, 1(2).
Ilhan, I., & Tezel, G. (2013). A genetic algorithm-support vector machine method with parameter optimization for selecting the tag SNPs. Journal of Biomedical Informatics, 46(2), 328–340. Retrieved from http://www.ncbi.nlm.nih.gov/pubmed/23262450
Jafari-Marandi, R., Davarzani, S., Soltanpour Gharibdousti, M., & Smith, B. K. (2018). An optimum ANN-based breast cancer diagnosis: Bridging gaps between ANN learning and decision-making goals. Applied Soft Computing Journal, 72, 108–120. https://doi.org/10.1016/j.asoc.2018.07.060
Kotu, V., & Deshpande, B. (n.d.). Predictive Analytics and Data Mining.
Levine, A. B., Schlosser, C., Grewal, J., Coope, R., Jones, S. J. M., & Yip, S. (2019). Rise of the Machines: Advances in Deep Learning for Cancer Diagnosis. Trends in Cancer, 5(3), 157–169. https://doi.org/10.1016/j.trecan.2019.02.002
Li, L., Yu, S., Xiao, W., Li, Y., Li, M., Huang, L., … Yang, H. (2014). Prediction of bacterial protein subcellular localization by incorporating various features into Chou’s PseAAC and a backward feature selection approach. Biochimie, 104(1), 100–107. https://doi.org/10.1016/j.biochi.2014.06.001
Meyer, H., Reudenbach, C., Hengl, T., Katurji, M., & Nauss, T. (2018). Improving performance of spatio-temporal machine learning models using forward feature selection and target-oriented validation. Environmental Modelling and Software, 101, 1–9. https://doi.org/10.1016/j.envsoft.2017.12.001
Nilashi, M., Ibrahim, O., Ahmadi, H., & Shahmoradi, L. (2017). A knowledge-based system for breast cancer classification using fuzzy logic method. Telematics and Informatics, 34(4), 133–144. https://doi.org/10.1016/j.tele.2017.01.007
Patrício, M., Pereira, J., Crisóstomo, J., Matafome, P., Gomes, M., Seiça, R., & Caramelo, F. (2018). Using Resistin, glucose, age and BMI to predict the presence of breast cancer. BMC Cancer, 18(1), 1–8. https://doi.org/10.1186/s12885-017-3877-1
Shukla, N., Hagenbuchner, M., Win, K. T., & Yang, J. (2018). Breast cancer data analysis for survivability studies and prediction. Computer Methods and Programs in Biomedicine, 155, 199–208. https://doi.org/10.1016/j.cmpb.2017.12.011
Singh, B. K. (2019). Determining relevant biomarkers for prediction of breast cancer using anthropometric and clinical features: A comparative investigation in machine learning paradigm. Biocybernetics and Biomedical Engineering, 39(2), 393–409. https://doi.org/10.1016/j.bbe.2019.03.001
Tapak, L., Shirmohammadi-Khorram, N., Amini, P., Alafchi, B., Hamidi, O., & Poorolajal, J. (2018). Prediction of survival and metastasis in breast cancer patients using machine learning classifiers. Clinical Epidemiology and Global Health. https://doi.org/10.1016/j.cegh.2018.10.003
Zhu, M., & Song, J. (2013). An embedded backward feature selection method for MCLP classification algorithm. Procedia Computer Science, 17, 1047–1054. https://doi.org/10.1016/j.procs.2013.05.133
DOI: https://doi.org/10.31294/infortech.v1i2.7398
DOI (PDF): https://doi.org/10.31294/infortech.v1i2.7398.g3791
Refbacks
- Saat ini tidak ada refbacks.
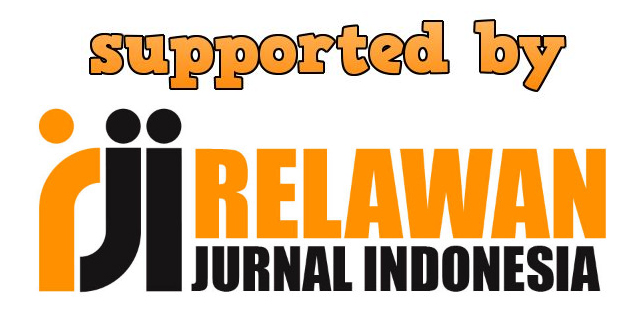
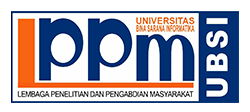