Prediksi Harga Komoditi Emas Menggunakan Metode Long Short-Term Memory Dengan Penambahan Optimalisasi
Sari
Teks Lengkap:
PDFReferensi
Bastian Sianturi, T., Cholissodin, I., & Yudistira, N. (2023). Penerapan Algoritma Long Short-Term Memory (LSTM) berbasis Multi Fungsi Aktivasi Terbobot dalam Prediksi Harga Ethereum. Jurnal Pengembangan Teknologi Informasi Dan Ilmu Komputer, 7(3), 1101–1107. http://j-ptiik.ub.ac.id
Chen, N. (2024). Exploring the development and application of LSTM variants. Applied and Computational Engineering, 53(1), 103–107. https://doi.org/10.54254/2755-2721/53/20241288
Dasari Siva Sankar, H. S. (2013). Gold Prices Prediction Using Random Forest. International Journal for Research in Applied Science & Engineering Technology (IJRASET), 11(V), 1–23.
Dr. S. Sasikala, D. R. B. (2024). An Enhanced Study on Gold Price Prognosis using Machine Learning. International Journal of Advanced Research in Science, Communication and Technology, 2024, p. 1-7. https://doi.org/https://doi.org/10.48175/ijarsct-18401
El-Rashidy, M. A. (2021). A novel system for fast and accurate decisions of gold-stock markets in the short-term prediction. Neural Comput & Applic. https://doi.org/https://doi.org/10.1007/s00521-020-05019-x
Ferdinandus, Y. R. M., Kusrini, K., & Hidayat, T. (2023). Gold Price Prediction Using the ARIMA and LSTM Models. Sinkron, 8(3), 1255–1264. https://doi.org/10.33395/sinkron.v8i3.12461
Hidayat, W. F., Julianto, M. F., Malau, Y., Setiadi, A., & Sriyadi. (2023). Implementation of LSTM and Adam Optimization as a Cryptocurrency Polygon Price Predictor. 2023 International Conference on Information Technology Research and Innovation, ICITRI 2023, 123–127. https://doi.org/10.1109/ICITRI59340.2023.10249571
Jevtić, A., Riznić, D., & Tomić, M. (2024). Gold price prediction based on the Monte Carlo method. XX(May), 201–209. https://doi.org/10.5937/imcsm24020j
K, D. S., B, S., Y, R., Shreya, A., & Kavitha, D. (2024). Data Mining Strategies for Gold Price Prediction Using Multi-Factorial Influences. International Journal For Multidisciplinary Research, 6(3), 1–15. https://doi.org/10.36948/ijfmr.2024.v06i03.18567
Mahajan, R., Patil, P., Chikmurge, D., & Barve, S. (2023). Forecasting Gold Price using Ensemble based Machine Learning Approach. 2023 International Conference on Innovative Computing, Intelligent Communication and Smart Electrical Systems (ICSES), 1–6. https://doi.org/10.1109/ICSES60034.2023.10465360
Manjula, K. A., & Karthikeyan, P. (2019). Gold price prediction using ensemble based machine learning techniques. Proceedings of the International Conference on Trends in Electronics and Informatics, ICOEI 2019, 2019-April(Icoei), 1360–1364.
Mustapha, A., Mohamed, L., & Ali, K. (2021). Comparative study of optimization techniques in deep learning: Application in the ophthalmology field. Journal of Physics: Conference Series, 1743(1). https://doi.org/10.1088/1742-6596/1743/1/012002
Nugroho, N. C. T., & Hidayat, E. Y. (2024). Implementation of Adam Optimizer using Recurrent Neural Network (RNN) Architecture for Diabetes Classification. Jurnal Media Informatika Budidarma, 8(1), 421–429. https://doi.org/10.30865/mib.v8i1.7254
Obayya, M., Maashi, M. S., Nemri, N., Mohsen, H., Motwakel, A., Osman, A. E., Alneil, A. A., & Alsaid, M. I. (2023). Hyperparameter Optimizer with Deep Learning-Based Decision-Support Systems for Histopathological Breast Cancer Diagnosis. Cancers, 15(3). https://doi.org/10.3390/cancers15030885
Salis, V. E., Kumari, A., & Singh, A. (2019). Prediction of Gold Stock Market Using Hybrid Approach. Emerging Research in Electronics, Computer Science and Technology. https://doi.org/10.1007/978-981-13-5802-9_70
Shanmugavadivu, P., Mary Shanthi Rani M, Chitra P, Lakshmanan S, Nagaraja P, & Vignesh U. (2022). Bio-Optimization of Deep Learning Network Architectures. Security and Communication Networks, 2022(ii). https://doi.org/10.1155/2022/3718340
Singh, N. (2024). Artificial Intelligence-Driven Model for Gold Price Prediction. Interantional Journal of Scientific Research in Engineering and Management, 08(05), 1–5. https://doi.org/10.55041/ijsrem33199
Tripurana, N., Kar, B., Chakravarty, S., Paikaray, B. K., & Satpathy, S. (2021). Gold Price Prediction Using Machine Learning Techniques. CEUR Workshop Proceedings, 3283, 274–281. https://doi.org/10.54097/gdm0kc53
Trivedi, U. B., Somvanshi, T. V. S., & J, S. P. (2022). Gold prices prediction: Comparative study of multiple forecasting models. YMER Digital, 21(07), 745–764. https://doi.org/10.37896/ymer21.07/60
Wang, H., Dong, X., Qu, H., Liao, J., & Ma, D. (2024). Gold Stock Price Forecasting Based on Nonlinear Weighted Particle Swarm (IPSO) Optimised Support Vector Machine (SVM) Time Series. Advances in Economics, Management and Political Sciences, 85(1), 118–124. https://doi.org/10.54254/2754-1169/85/20240857
Yi, D., Ahn, J., & Ji, S. (2020). An effective optimization method for machine learning based on ADAM. Applied Sciences (Switzerland), 10(3). https://doi.org/10.3390/app10031073
DOI: https://doi.org/10.31294/infortech.v6i2.24440
DOI (PDF): https://doi.org/10.31294/infortech.v6i2.24440.g6676
Refbacks
- Saat ini tidak ada refbacks.
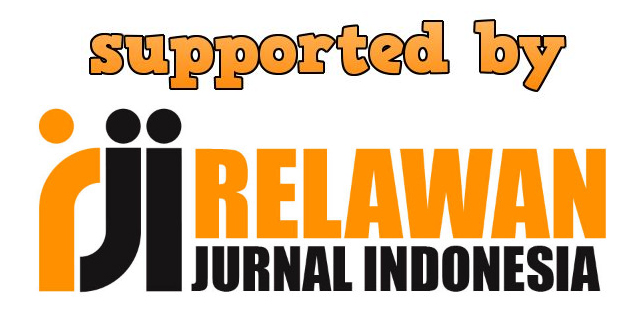
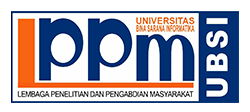