Pengaruh Principal Component Analysis Pada Naïve Bayes dan K-Nearest Neighbor Untuk Prediksi Dini Diabetes Melitus Menggunakan Rapidminer
Abstract
Keywords: Kelompok; DiabetesMelitus; Naïve Bayes; k-Nearest Neighbor; PCA.
Abstract
Patients with diabetes mellitus experience disturbances in the metabolic system caused by the pancreas not producing insulin or using insulin in metabolism that is not effective more and more. Concern for healthy living has decreased drastically, so the spike in deaths from this disease is high. Many people do not understand the early symptoms that appear, making it difficult to recover. This is because there is no early prediction of sufferers of the disease. This study explains the effect of principal component analysis (PCA) to find optimal features in the classification of early prediction of diabetes mellitus in naïve Bayers and k-nearest neighbors plus the open rapidminer application that can be used as a test tool for data accuracy. The research material used comes from the Learning Repository Early Stage Diabetes Risk Prediction Dataset from the Kaggle website, namely diabet_data_upload.csv. The number of records used is 520 rows of data and 17 table names for each existing row of data. The purpose of using the two grouping methods is to show the most accurate accuracy of the processed data. The results of the study provide a study that the k-nearest-neighbor formula with principal component analysis can work better than just k-nearest-neighbor. The performance of k-nearest neighbor with principal component analysis (PCA) is better with an accuracy value of 93.27%, while the accuracy without principal component analysis in this case only uses the k-nearest-neighbor algorithm is only 90.70. These results are obtained by considering the existing records and the value of k = 5, then the result is that the k-nearest neighbor algorithm uses the principal component analysis (PCA) method to classify diabetes diagnoses as high. Exact value result..
Keywords: Group; Diabetes Mellitus; Naïve Bayes; k-Nearest Neighbor; PCA.
Full Text:
PDF (1-12)References
Ardiyansyah, Panny Agustia Rahayuningsih, and Reza Maulana. 2018. “Analisis Perbandingan Algoritma Klasifikasi Data Mining Untuk Dataset Blogger Dengan Rapid Miner.” Jurnal Khatulistiwa Informatika VI(1):20–28.
Fernanda IS, Ratnawati ED, Adikara PP (2017) Identifikasi Penyakit Diabetes Mellitus Menggunakan Metode Modified KNearest Neighbor (MKNN), Jurnal Pengembangan Teknologi Informasi dan Ilmu Komputer e-ISSN: 2548-964X Vol. 1, No. 6, Juni 2017, hlm. 507-513 ← Journal
S. E. Viswapriyaa, (2019). International Journal of Engineering Research & Technology (IJERT) ISSN: 2278-0181 IJERTV8IS100318 (This work is licensed under a Creative Commons Attribution 4.0 International License.) Published by : www.ijert.org Vol. 8 Issue 10, October-2019 . ← Journal
Novianto Dian, Sugihartono Tri (2020). Sistem Deteksi Kualitas Buah Jambu Air Berdasarkan Warna Kulit Menggunakan Algoritma Principal Component Analysis (Pca) dan K-Nearest Neigbor (K-NN), Jurnal Ilmiah Informatika Global Volume 11 No. 2 Desember 2020 ISSN PRINT : 2302-500X ISSN ONLINE : 2477-3786← Journal
Ramadhani AR, Niswatin KR (2018). Sistem Diagnosa Diabetes Menggunakan Metode K-NN Jurnal Sains dan Informatika p-ISSN: 2460-173X Volume 4, Nomor 2, November 2018 e-ISSN: 2598-58414079(200202)37:1%3C51::AID-CRAT51%3E3.0.CO;2-N ←Journal
Li, M., Xing, S., Yang, L., Fu, J., Lv, P., Wang, Z., Yuan, Z. (2019). Nickel-loaded ZSM-5 catalysed hydrogenation of oleic acid: The game between acid sites and metal centres. Applied Catalysis A: General. 587, 117112. DOI: 10.1016/j.apcata.2019.117112. ←Journal
Natasuwarna, A.P., 2019, Data Mining dengan Penerapan Aplikasi RapidMiner, Pustakaone, Jogjakarta
Argina MA (2020) Penerapan Metode Klasifikasi K-Nearest Neigbor pada Dataset Penderita Penyakit Diabetes Indonesian Journal of Data and Science ISSN: 2715-9930 ←Journal
Singh KH, Vishnavat K, R. Srinivasan (2018) Employee Performance And Leave Management Using Data Minning Technique. International Journal of Pure and Applied Mathematics Volume 118 No. 20 2018, 2063-2069 ISSN: 1311-8080 (printed version); ISSN: 1314-3395 ← Journal
Adebayo OA , Chaubey SM (2019). Data Minning Classification Technique On The Analysis Of Students Performance., GSJ: Volume 7, Issue 4, April 2019, Online: ISSN 2320-9186 ←Journal
Yahya, Gunawan Indra, Harianto Bambang (2017). Penerapan PCA dan K-NN Untuk Meningkatkan Nilai Akurasi Pengenalan Wajah. JURNAL INFORMATIKA HAMZANWADI Vol. 2 No. 1, Mei 2017, hal. 81-90 ISSN: 2527 - 6069 ←Journal
BN Azmi, A. Hermawan, D. Avianto (2020). Analisis Pengaruh PCA Pada Klasifikasi Kualitas Air Menggunakan Algoritma K-Nearest Neighbor dan Logistic Regression. ←Journal
Ridwan Achmad (2020) Penerapan Algoritma Naïve Bayes Untuk Klasifikasi Penyakit Diabetes Mellitus ←Journal
Putry M. N, Sari NB M.Kom (2022) Komparasi Algoritma KNN dan Naïve Bayes Untuk Klasifikasi Diagnosis Penyakit Diabetes Melitus ←Journal
A. Naik and L. Samant, “Correlation Review of Classification Algorithm Using Data Mining Tool: WEKA, Rapidminer, Tanagra, Orange and Knime,” Procedia Comput. Sci.,vol. 85, pp. 662–668, 2016, doi: 0.1016/j.procs.2016.05.251.
Hermawan Arief, Wibowo Adityo Permana, Wijaya A Setiawan, (2022) The Improvement of Artificial Neural Network Accuracy Using Principle Component Analysis Approach←Jurnal
Krismawan DA, Rachmawanto EH, (2022) Principal Component Analysis (PCA) dan K-nearest Neighbor (KNN) dalam Deteksi Masker Pada Wajah. ←Journal
DOI: https://doi.org/10.31294/evolusi.v11i1.14728
ISSN: 2657-0793 (online). ISSN: 2338-8161 (print)
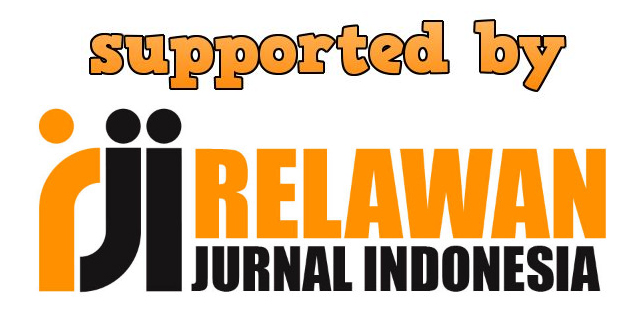
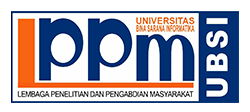